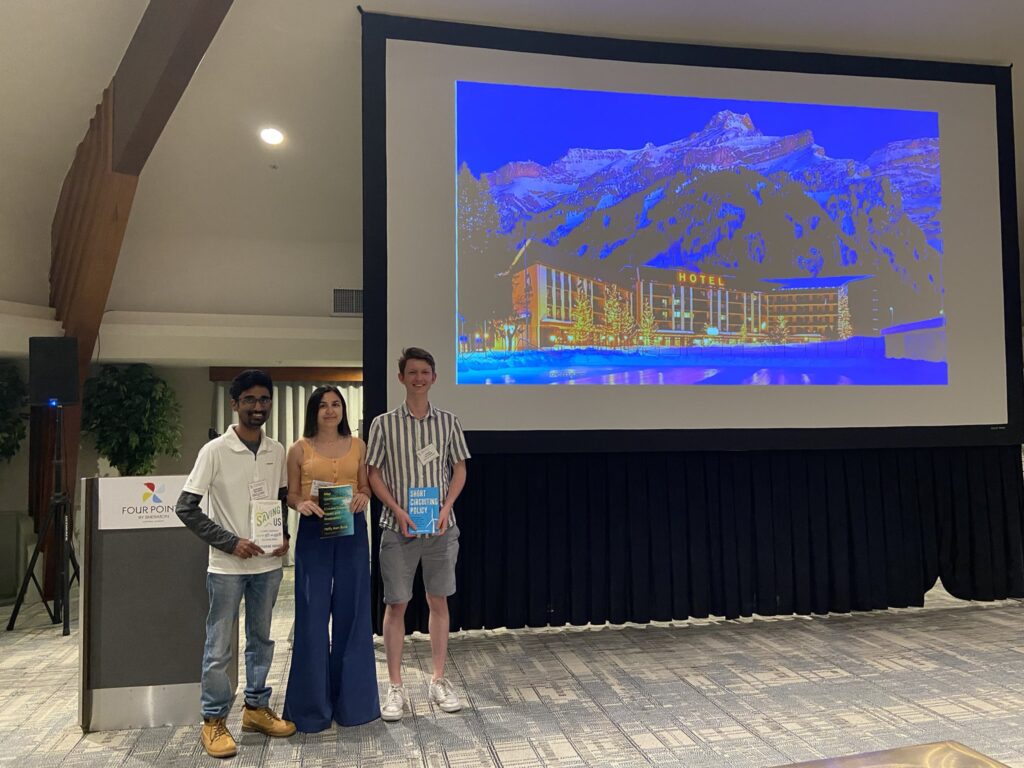
Fast-track development of carbon capture: Integration of materials, processes, techno-economics, and life-cycle assessment
Finding Promising Carbon Capture Adsorbents Through a Diversity-Driven Search
”This study focuses on developing a diverse database of metal-organic frameworks (MOFs) for high throughput computational screening. Material design, molecular simulation and machine learning techniques were used to generate and evaluate these MOFs for post-combustion carbon capture. We find that many of the MOFs from our database perform better than Zeolite-13X , a benchmark material for post-combustion carbon capture. Read more here
Screening MOF databases using machine learning: Making the link between material properties and their performance
There are millions of porous materials that have the ability to remove carbon dioxide from a range of sources. A particularly interesting class of material are Metal-Organic Frameworks (MOFs) which are often used in adsorption-based processes due to their tailorable properties. In-silico databases have been developed in an effort to find MOFs which are suitable for a specific task, such as carbon dioxide separation. However, screening these large databases is computationally heavy. Machine learning (ML) techniques hold a powerful role in screening studies and have many uses. In particular, ML based surrogate models can provide quick estimates of computationally heavy calculations (i.e. process models), and can allow quick and accurate searching of databases. This poster details the application of machine learning to a MOF screening study: removing methane from a dilute stream, such as the case in ventilated air methane processing which is a large greenhouse contributor from the mining industry. The screening workflow consists of isotherm generation and a temperature vacuum swing adsorption process model. Data is collected and ML surrogate models are developed. This is a case study for a screening methodology, with the aim of applying this to carbon capture processes.